Addressing Challenges In Automated Visual Inspection Of Lyophilized Vials
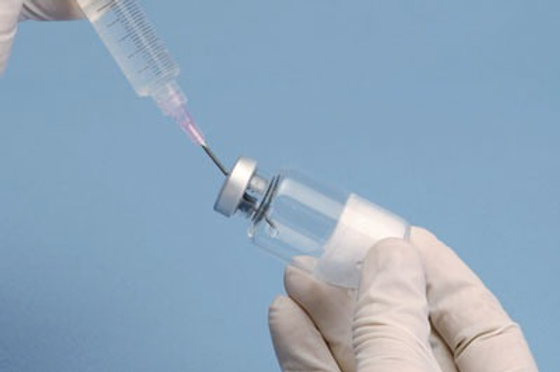
Table of Contents
Image Acquisition and Preprocessing Challenges
The first major hurdle in automated visual inspection lies in acquiring high-quality images suitable for reliable defect detection. Several factors complicate this process.
Lighting and Shadow Effects
Achieving consistent and uniform illumination is crucial. Shadows and reflections can easily obscure defects, leading to false negatives. Careful control of lighting is paramount.
- Diffused lighting: Soft, diffused light minimizes harsh shadows.
- Multi-angle illumination: Using light sources from multiple angles can help reveal defects hidden in shadows.
- Polarization filters: These filters can reduce glare and reflections from vial surfaces.
Variations in lighting intensity or angle can drastically affect the appearance of the lyophilized cake and vial, potentially leading to both false positives (flagging non-defective vials) and false negatives (missing actual defects) in automated defect detection.
Vial Orientation and Positioning
Inconsistent vial orientation during the inspection process significantly impacts the accuracy of automated systems. Precise and consistent presentation to the camera is essential.
- Robotic systems: Automated robotic systems offer precise vial handling and positioning, ensuring consistent orientation.
- Consistent presentation: Careful design of the vial handling and presentation mechanism is crucial to maintain consistent orientation.
Variations in vial shape, size, and the presence of any irregularities can further complicate accurate positioning, highlighting the need for adaptable robotic systems and sophisticated image processing algorithms to compensate for these variations.
Background Noise and Artifact Reduction
Background noise and artifacts in the acquired images (e.g., dust particles, scratches on the conveyor belt) interfere with accurate defect detection.
- Image filtering: Various filtering techniques can effectively remove noise and artifacts.
- Image segmentation: This technique isolates the vial from the background, reducing the impact of noise.
- Noise reduction algorithms: Advanced algorithms, such as wavelet denoising or anisotropic diffusion, can effectively suppress noise while preserving important details.
The choice of algorithm often depends on the specific type of noise and artifacts present in the images. For example, Gaussian noise may require different processing than salt-and-pepper noise.
Defect Detection and Classification Challenges
Identifying defects in lyophilized vials is inherently challenging due to the subtle nature of many defects and the variations in the appearance of the lyophilized cake.
Subtle Defect Identification
Detecting subtle defects such as cracks, particulate matter, and inconsistencies in the lyophilized cake requires advanced image analysis techniques.
- Machine learning (ML): ML algorithms can learn to recognize patterns associated with defects from labeled training data.
- Deep learning (DL): DL, a subset of ML, excels at identifying complex patterns and subtle variations, improving defect detection sensitivity.
Training these algorithms requires large, carefully annotated datasets representing a wide range of defects and variations in lyophilized cake appearance. This is a crucial and time-consuming step in developing a robust automated visual inspection system.
False Positive and False Negative Minimization
Minimizing both false positives and false negatives is critical for maintaining high product quality and minimizing waste.
- Validation datasets: Independent datasets are used to evaluate the system's performance and identify areas for improvement.
- Performance metrics: Metrics such as precision, recall, and the F1-score quantify the system's accuracy.
False positives lead to unnecessary rejection of good vials, increasing costs and reducing throughput. False negatives, however, are far more dangerous, as they allow defective vials to reach the market, potentially harming patients.
Dealing with Variations in Lyophilized Cake Appearance
Variations in the appearance of the lyophilized cake, such as color, texture, and density, can significantly affect defect detection accuracy.
- Robust algorithms: Algorithms must be designed to account for natural variations while reliably detecting actual defects.
- Standardization: Standardizing lyophilization processes can minimize variations in cake appearance, improving the accuracy of automated inspection.
By carefully controlling the lyophilization parameters and employing robust image analysis techniques, it’s possible to minimize the impact of these variations.
System Integration and Validation Challenges
Integrating and validating automated visual inspection systems within existing production lines pose significant logistical and regulatory challenges.
Integration with Existing Production Lines
Integrating the automated system seamlessly into existing lyophilization and packaging lines requires careful planning.
- Throughput: The system must match or exceed the throughput of the existing line.
- Space constraints: Available space in the production facility must be considered.
- Equipment compatibility: The system must be compatible with existing equipment.
Successful integration requires close collaboration between equipment vendors, automation engineers, and production personnel.
Validation and Regulatory Compliance
Automated visual inspection systems must be rigorously validated to meet regulatory standards, such as FDA 21 CFR Part 11.
- Comprehensive documentation: Detailed documentation of the system's design, testing, and performance is essential.
- Testing and qualification: Thorough testing procedures must demonstrate the system's accuracy, reliability, and traceability.
Meeting regulatory requirements is crucial for ensuring the system's reliability and for obtaining regulatory approvals.
Conclusion: Overcoming Challenges in Automated Visual Inspection of Lyophilized Vials
Implementing effective automated visual inspection of lyophilized vials requires addressing various challenges related to image acquisition, defect detection, and system integration. Advanced image processing techniques, machine learning, and rigorous system validation are key to overcoming these hurdles. By leveraging these technologies and carefully planning integration, pharmaceutical manufacturers can significantly improve the efficiency and accuracy of their visual inspection processes, ultimately enhancing product quality and patient safety. Contact our experts today to discuss how we can help you optimize your automated visual inspection processes and improve your product quality.
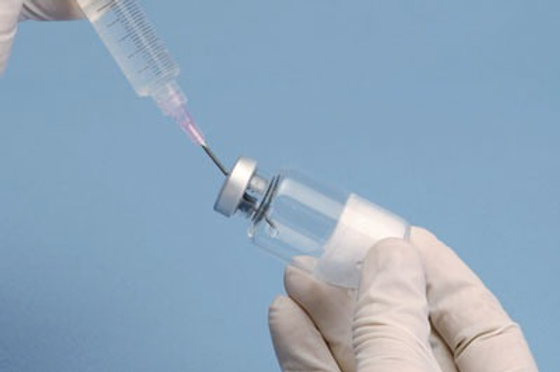
Featured Posts
-
How Trumps Presidency Will Shape Zuckerbergs Leadership At Meta
May 11, 2025 -
2025 Indy 500 Which Driver Wont Be Racing
May 11, 2025 -
Payton Pritchard Celtics Guard Inks Shoe Deal With Converse
May 11, 2025 -
Sea Level Rise Urgent Action Needed To Protect Coastal Regions
May 11, 2025 -
Relegation Battle Holstein Kiel Earns Crucial Draw Against Champions League Chasing Mainz
May 11, 2025
Latest Posts
-
Canada Us Tariffs A Partial Removal
May 12, 2025 -
Final American Hostage Released By Hamas In Gaza
May 12, 2025 -
India Pakistan Ceasefire Report Of Five Indian Soldier Deaths
May 12, 2025 -
Understanding The Value Of Middle Management In Todays Business Environment
May 12, 2025 -
The Edan Alexander Hostage Case Latest Developments And Release Hopes
May 12, 2025