AI-Driven Drug Discovery: D-Wave's Quantum Computing Platform (QBTS)
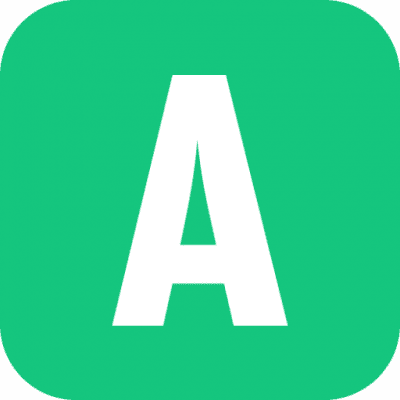
Table of Contents
How D-Wave's QBTS Accelerates Drug Discovery
D-Wave's QBTS leverages the power of quantum annealing, a unique quantum computing approach, to tackle complex computational problems far exceeding the capabilities of classical computers. Quantum annealing excels at finding optimal solutions within vast search spaces, a characteristic particularly beneficial for drug discovery. The sheer size and complexity of datasets involved in molecular simulations and drug interactions are perfectly suited to this technology. QBTS allows for:
- Faster identification of potential drug candidates: By rapidly analyzing massive molecular datasets, QBTS can pinpoint promising molecules much faster than traditional methods.
- Improved prediction of drug efficacy and safety: Quantum simulations can accurately model drug-target interactions, predicting a drug's effectiveness and potential side effects with greater precision.
- Reduced time and cost associated with preclinical trials: More accurate predictions from QBTS can significantly streamline the preclinical phase, saving valuable time and resources.
- Optimization of drug design parameters: QBTS can optimize various parameters like dosage, delivery methods, and formulation to enhance drug efficacy and minimize adverse effects. This leads to better-designed drugs from the outset.
These advantages translate to a significantly faster and more efficient accelerated drug discovery process, ultimately bringing life-changing medications to patients sooner. The use of molecular simulation within QBTS provides an unparalleled level of detail and accuracy, surpassing the limitations of classical computational methods.
The Role of AI in D-Wave's Quantum Drug Discovery Approach
The synergy between AI algorithms and quantum computing is crucial to D-Wave's approach. AI acts as a powerful complement to QBTS, enhancing its capabilities in several key areas:
- AI for feature selection and data preprocessing for quantum algorithms: AI algorithms can intelligently select the most relevant data features from massive datasets, preparing them optimally for quantum computations. This crucial preprocessing step significantly enhances the efficiency and accuracy of the quantum calculations.
- AI for post-processing and interpretation of quantum computation results: The results from quantum computations are often complex and require sophisticated analysis. AI algorithms can efficiently process and interpret these results, extracting meaningful insights for drug development.
- AI for designing efficient quantum algorithms for drug discovery problems: AI can assist in designing and optimizing quantum algorithms specifically tailored to address unique drug discovery challenges, further boosting the efficiency of the QBTS platform. This includes optimizing the quantum annealing process for specific molecular simulations.
This intelligent interplay between AI and quantum computing represents a paradigm shift in drug candidate identification and significantly enhances the overall effectiveness of the AI-driven drug discovery process. The use of machine learning within this process allows for continuous improvement and adaptation based on the data generated.
Case Studies and Real-World Applications of QBTS in Drug Discovery
While specific details of ongoing projects may be confidential due to competitive reasons, D-Wave has collaborated on projects that showcase the potential of QBTS. For example, research is ongoing into utilizing the platform to accelerate the discovery of new antibiotics. The hope is to significantly decrease the time it takes to identify and test potential compounds. Initial results hint at enhanced speed and efficiency compared to traditional methods. Furthermore, explorations are underway in applying QBTS to optimizing the design of existing drugs for improved efficacy and reduced side effects. These ongoing endeavors highlight the transformative potential of QBTS in pharmaceutical research. As research continues, we can expect to see more compelling case studies demonstrating the real-world impact of this powerful technology in the years to come.
Challenges and Future Directions of AI-Driven Drug Discovery with QBTS
Despite its immense potential, the integration of AI and quantum computing in drug discovery presents certain challenges:
- Scaling up quantum computations for larger datasets: While QBTS is powerful, handling extremely large datasets still presents computational challenges. Future developments will need to focus on scaling up the quantum computing resources to match the ever-increasing data sizes in drug discovery.
- Improving the accuracy and reliability of quantum simulations: Ongoing research aims to improve the precision and reliability of quantum simulations to ensure the accuracy of predictions.
- Developing more sophisticated AI algorithms for drug discovery: Advancements in AI are needed to develop more effective algorithms specifically tailored for the intricacies of drug discovery problems.
- Integration with other advanced technologies (e.g., high-throughput screening): Integrating QBTS with other advanced technologies, such as high-throughput screening, will further enhance its capabilities and streamline the overall drug development workflow.
The future of QBTS in drug development looks bright. As technology progresses, we can anticipate even more efficient and accurate AI-driven drug discovery fueled by quantum computing, leading to faster development timelines and ultimately, better treatments for patients worldwide.
Conclusion: The Future of Drug Discovery Lies in AI and Quantum Computing
The integration of D-Wave's QBTS with AI offers a transformative approach to accelerated drug discovery. This powerful combination overcomes many limitations of traditional drug development, promising faster, cheaper, and more effective ways to bring life-saving medications to market. The potential for revolutionizing the future of drug development through AI-driven drug discovery and quantum annealing is undeniable. To learn more about how D-Wave's QBTS can accelerate your drug discovery efforts, visit the D-Wave website today. The future of drug discovery is here, and it's powered by the convergence of AI and quantum computing.
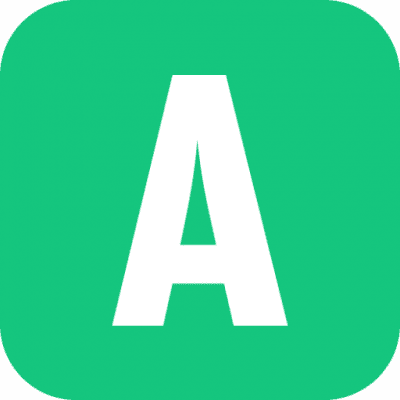
Featured Posts
-
Miami Hedge Fund Manager Banned From Us For Alleged Immigration Fraud
May 20, 2025 -
Festival Da Cunha Entrada Gratuita 90 Com Maiara E Maraisa Em Manaus
May 20, 2025 -
Diskussion Hamilton Och Leclercs Diskvalificering I F1
May 20, 2025 -
Ohio Derailment Investigation Into Lingering Toxic Chemicals In Buildings
May 20, 2025 -
Jacob Friisin Avauskokoonpano Yllaetys Glen Kamara Ja Teemu Pukki Sivussa
May 20, 2025
Latest Posts
-
Poy Tha Vro Efimereyonta Giatro Stin Patra 10 And 11 Maioy
May 20, 2025 -
Dimotiko Odeio Rodoy Synaylia Ton Kathigiton Stin Dimokratiki
May 20, 2025 -
Odigos Gia Efimereyontes Iatroys Stin Patra 10 11 5
May 20, 2025 -
Kathigites Dimotikoy Odeioy Rodoy Synaylia Stin Dimokratiki
May 20, 2025 -
Katalogos Efimereyonton Giatron Patras 10 And 11 Maioy
May 20, 2025