Building Ethical AI: A Conversation With Microsoft's Design Leader
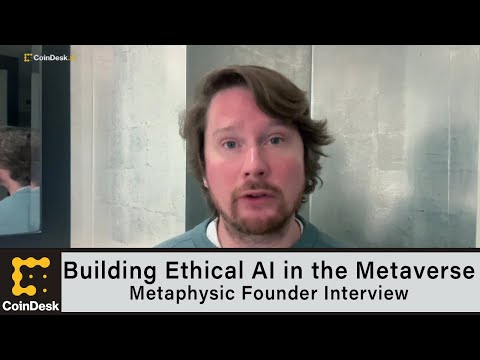
Table of Contents
Defining Ethical AI Principles
Ethical AI refers to the development and deployment of AI systems that align with human values and societal well-being. Its core principles are multifaceted and interconnected, encompassing fairness, transparency, accountability, privacy, robustness, and security. Let's examine each:
-
Fairness in AI: This means avoiding bias in algorithms and data sets. AI systems should treat all individuals equitably, regardless of race, gender, religion, or other protected characteristics. Unfair bias can lead to discriminatory outcomes, perpetuating existing inequalities.
-
Transparency and Explainability: Understanding how AI systems arrive at their decisions is crucial for building trust and ensuring accountability. Explainable AI (XAI) aims to make the decision-making processes of AI models more transparent and understandable.
-
Accountability and Responsibility: Clear lines of responsibility must be established for the development, deployment, and consequences of AI systems. Who is accountable when an AI system makes a mistake or causes harm? This requires careful consideration of legal and ethical frameworks.
-
Robustness and Security: Ethical AI systems must be resilient to attacks and misuse. They should be designed to withstand malicious attempts to manipulate their outputs or compromise their integrity. Security vulnerabilities can have serious consequences.
-
User Privacy: AI systems often rely on vast amounts of data, raising concerns about user privacy. Ethical AI development requires robust data protection measures to ensure user privacy is respected and protected.
Microsoft's Approach to Ethical AI Development
Microsoft has taken a proactive approach to integrating ethical considerations into its AI development lifecycle. Their commitment to responsible innovation is evident in their internal guidelines and initiatives.
-
Internal Frameworks and Tools: Microsoft employs various internal frameworks and tools to guide ethical AI development. These might include automated bias detection tools, ethical review boards for AI projects, and comprehensive guidelines on data privacy and security.
-
Commitment to Responsible Innovation: Microsoft's commitment goes beyond simply adhering to regulations. They actively invest in research and development focused on mitigating AI risks and promoting responsible use of AI technologies.
-
Case Studies and Examples: While specific details may be confidential, Microsoft likely has internal examples of how their ethical AI guidelines have been applied in developing products and services. These cases could illustrate how the principles discussed earlier are translated into practice. They might include projects that prioritized fairness in loan applications, ensuring transparency in facial recognition, or implementing robust security measures in their cloud-based AI services.
Addressing Bias and Fairness in AI Systems
One of the most significant challenges in ethical AI is addressing bias. Bias can creep into AI systems at various stages, from data collection and preprocessing to algorithm design and deployment.
-
Bias Detection and Reduction: Techniques like data augmentation, re-weighting, and adversarial training are used to identify and mitigate bias in training data. Careful data curation and preprocessing are essential steps to minimize bias from the start.
-
Fairness and Inclusivity in AI Outputs: Ensuring fairness requires continuous monitoring and evaluation of AI systems’ performance across different demographic groups. This might involve using fairness metrics to assess the impact of AI decisions on various populations.
-
Role of Diverse Teams: Diverse development teams, representing a range of backgrounds and perspectives, are crucial for identifying and addressing potential biases in AI systems. A diverse team is more likely to anticipate and mitigate unintended consequences.
The Future of Ethical AI Design and Collaboration
The field of ethical AI is constantly evolving. New challenges and opportunities emerge as AI technology advances.
-
Industry Collaboration and Standardization: Collaboration between AI developers, researchers, policymakers, and ethicists is vital to establish shared standards and best practices for ethical AI development. Industry-wide initiatives and standardization efforts can help to ensure consistency and accountability.
-
Regulation and Governance: Effective regulation and governance frameworks are needed to ensure responsible AI development and deployment. This might involve creating guidelines for data privacy, algorithmic transparency, and accountability for AI-related harms.
-
Future Challenges and Opportunities: The future of ethical AI presents both exciting opportunities and significant challenges. Addressing issues such as the explainability of complex AI models, the potential for AI misuse, and the societal impact of widespread AI adoption will require ongoing research, collaboration, and thoughtful consideration.
Conclusion
This hypothetical conversation with a Microsoft design leader has highlighted the critical aspects of building ethical AI. From defining core principles like fairness, transparency, and accountability, to tackling bias and fostering collaboration across the industry, the path towards responsible AI development requires a multifaceted and ongoing approach. Microsoft's commitment to these principles provides a valuable example for other organizations. Building ethical AI is not merely a technological challenge, but a societal imperative. To learn more about ethical AI best practices and responsible innovation, explore Microsoft's resources (and other leading organizations) and join the conversation on building a more ethical and equitable future with AI. Let's work together to build a future powered by ethical AI.
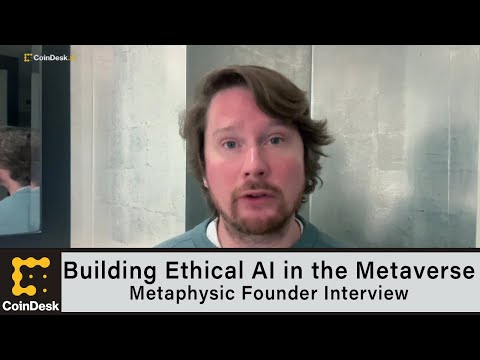
Featured Posts
-
Understanding The Value Of Middle Managers Benefits For Companies And Their Workforces
Apr 26, 2025 -
Millions In Losses Office365 Executive Inboxes Breached Criminal Charges Filed
Apr 26, 2025 -
Stock Market Valuations Bof A Says Dont Panic
Apr 26, 2025 -
Ukraines Nato Aspiration Trumps Perspective And Implications
Apr 26, 2025 -
Cassidy Hutchinsons Fall Memoir Insights From A Key Jan 6 Player
Apr 26, 2025
Latest Posts
-
Edmontons Nordic Spa Development Receives Council Approval
May 10, 2025 -
Young Thugs Uy Scuti When Can We Expect The New Album
May 10, 2025 -
Uy Scuti Album Young Thugs Upcoming Release Date Speculation
May 10, 2025 -
Young Thugs Uy Scuti Release Date Hints And Fan Speculation
May 10, 2025 -
Uy Scuti Album Young Thugs Upcoming Project And Potential Release Timeline
May 10, 2025