Mining Meaning From Mess: An AI's Approach To Creating A Podcast From Repetitive Scatological Documents
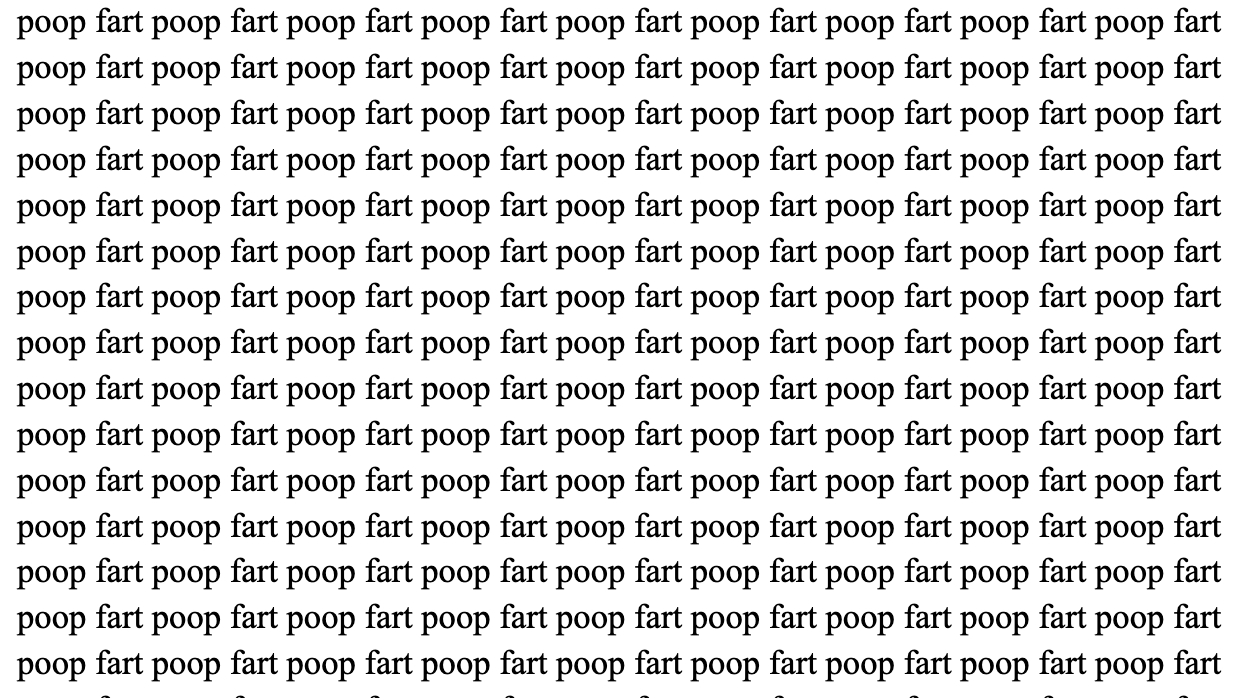
Table of Contents
The sheer volume of repetitive, seemingly useless data—like scatological records—often overwhelms researchers. But what if this "mess" held hidden narratives, untold stories waiting to be discovered? This article explores how Artificial Intelligence (AI) can mine meaning from messy, repetitive scatological documents, transforming them into engaging and informative podcasts. We'll delve into the process, from data cleaning and initial analysis to audio production and final distribution, showcasing how AI can revolutionize how we approach and understand complex datasets.
Data Preprocessing and Analysis: Laying the Foundation for a Meaningful Podcast
Before we can even think about creating an engaging podcast, the raw scatological data needs careful preparation. This crucial preprocessing stage ensures the AI can effectively analyze the information and extract meaningful insights.
Cleaning the Data:
Addressing inconsistencies and noise in the raw scatological data is crucial for accurate analysis. This involves several key steps:
- Removing irrelevant entries and duplicates: Identifying and eliminating entries that don't contribute to the research goals is essential for efficient data processing. This might include removing entries with incomplete information or those clearly outside the defined parameters of the study.
- Handling missing data through imputation techniques: Missing data points are a common problem. AI can employ various imputation techniques, such as mean/median imputation or more sophisticated methods like K-Nearest Neighbors, to fill in the gaps while minimizing bias.
- Standardizing data formats and units: Ensuring consistency in data formats (dates, measurements, etc.) and units is vital for accurate analysis. This might involve converting different date formats to a single standard or harmonizing different units of measurement.
Identifying Patterns and Trends:
Once the data is clean, AI algorithms can reveal underlying patterns and trends often invisible to the human eye. This is where the real power of AI comes into play:
- Clustering similar data points to identify key themes or trends: Unsupervised machine learning techniques, such as K-means clustering or hierarchical clustering, can group similar data points together, revealing hidden themes and patterns within the scatological data.
- Time-series analysis to detect changes over time: If the data is collected over time, time-series analysis can identify trends, seasonality, and other temporal patterns. This could reveal interesting correlations between scatological data and other factors.
- Statistical analysis to identify significant correlations: Statistical methods, aided by AI, can identify significant correlations between different variables within the dataset, providing valuable insights for narrative development.
AI-Powered Transcription and Content Creation: Transforming Data into Narrative
With the data preprocessed and analyzed, the next step is to transform the raw information into a compelling narrative suitable for a podcast.
Automated Transcription:
AI transcription services are instrumental in converting the raw scatological data into a usable text format.
- Selecting an appropriate AI transcription service based on accuracy and cost: Several services offer varying levels of accuracy and pricing. Choosing the right service depends on budget and the required level of accuracy. Consider factors like speaker diarization capabilities if multiple individuals are involved in the data.
- Reviewing and correcting transcription errors manually: While AI transcription is highly accurate, manual review and correction are essential to ensure the final transcript is free of errors and suitable for narrative development.
Narrative Generation:
AI can assist in structuring the transcribed data into a coherent and engaging narrative.
- Using natural language processing (NLP) to identify key insights and create talking points: NLP techniques can help identify the most significant findings from the data analysis and formulate key talking points for the podcast.
- Employing AI story generation tools to craft a compelling narrative arc: AI tools can help structure the narrative, creating a compelling arc with a beginning, middle, and end. However, human oversight is crucial to maintain accuracy and coherence.
- Incorporating human oversight to ensure accuracy and narrative coherence: While AI can generate narratives, human input ensures the final product accurately reflects the data and maintains a clear, logical flow.
Audio Production and Podcast Distribution: Bringing the Story to Life
The final stage involves bringing the narrative to life through audio production and distribution.
Voice Generation:
AI text-to-speech (TTS) technology is a powerful tool for podcast narration.
- Choosing a TTS voice that matches the intended tone and audience: The choice of voice significantly impacts listener engagement. Selecting a voice that aligns with the podcast's tone and target audience is crucial.
- Adding background music and sound effects to enhance listener engagement: Music and sound effects can significantly improve the listening experience, adding emotional depth and enhancing the narrative.
Podcast Distribution:
Effective distribution ensures your podcast reaches the target audience.
- Optimizing the podcast metadata (title, description, keywords) for search engines: Using relevant keywords in the podcast title, description, and tags improves discoverability through search engines.
- Submitting the podcast to directories like Apple Podcasts, Spotify, and Google Podcasts: Submitting your podcast to popular directories ensures maximum accessibility to potential listeners.
Conclusion:
Mining meaning from messy, repetitive data like scatological documents is achievable with the power of AI. By utilizing AI-powered tools for data processing, transcription, content creation, and audio production, researchers can transform seemingly useless information into engaging and informative podcasts. This approach opens new avenues for research communication and public engagement. Don't let your repetitive scatological data remain a mess—start mining meaning today! Explore the possibilities of AI-powered podcast creation and unlock the hidden stories within your data.
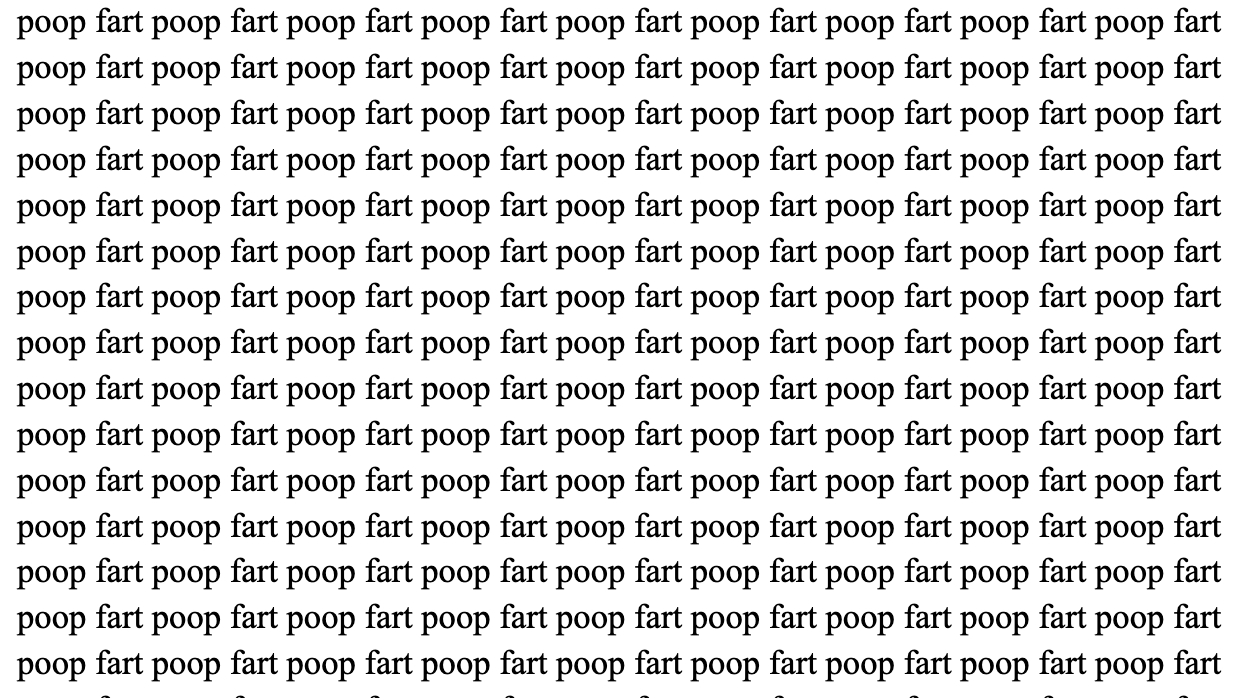
Featured Posts
-
Rediscovering Cannes Fun Crazy And Ludicrous Images From The Pre Digital Age
May 18, 2025 -
Why India Is Avoiding Trade With Pakistan Turkey And Azerbaijan
May 18, 2025 -
Teylor Svift Rekordnye Prodazhi Vinila Za Poslednee Desyatiletie
May 18, 2025 -
Los Angeles Palisades Fire Impact On Celebrity Homes A Comprehensive List
May 18, 2025 -
High Rated Netflix Romance Drama Surpassed By True Crime Docuseries
May 18, 2025
Latest Posts
-
Amanda Bynes Public Struggles And Her Path To Recovery
May 18, 2025 -
Two Run Blast Shohei Ohtanis Return And Rise In Japan
May 18, 2025 -
Gonsolins Solid Performance Leads Dodgers To Fifth Straight Victory
May 18, 2025 -
Gonsolins Impressive Return Fuels Dodgers Five Game Winning Streak
May 18, 2025 -
Confortos First Dodgers Home Run Powers 6 4 Win Over Mariners
May 18, 2025