Overcoming Hurdles In Automated Visual Inspection Of Lyophilized Drug Products
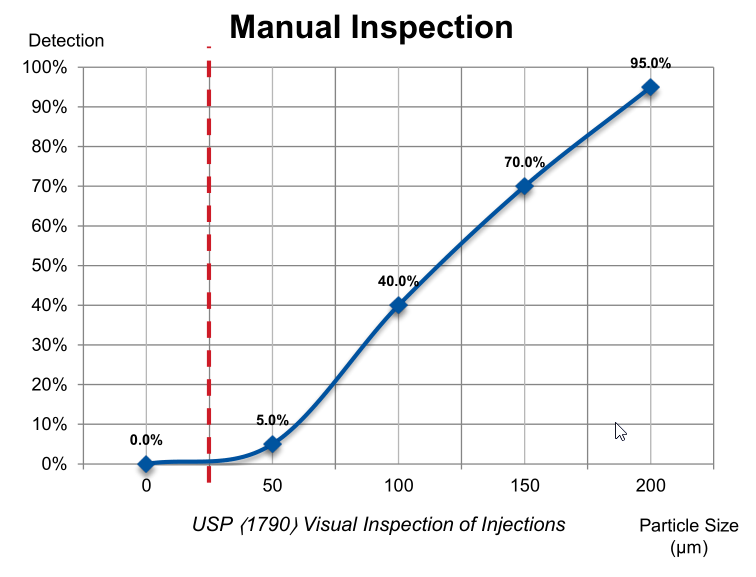
Table of Contents
Challenges in Image Acquisition for Lyophilized Drug Products
High-quality image acquisition is the cornerstone of effective automated visual inspection. However, several factors complicate image acquisition for lyophilized drug products, impacting the overall accuracy of defect detection. Key challenges include:
- Variations in vial shape and size: Inconsistencies in vial dimensions lead to variations in lighting, focus, and overall image quality, making consistent defect detection challenging. Standardized vial sizes and shapes would significantly improve image acquisition consistency.
- Surface reflections and glare: The glass vials and the lyophilized cake itself can cause significant reflections and glare, obscuring subtle defects like cracks or discolorations. Advanced lighting techniques, such as structured lighting or polarized light, can help mitigate this issue. Careful selection of lighting angles and intensities is also critical for optimal image acquisition.
- Achieving optimal lighting conditions: Consistent and defect-revealing images depend heavily on precise lighting. Achieving uniform illumination across all vials is challenging, particularly with variations in vial positioning and orientation within the inspection system. Implementing advanced lighting control systems and sophisticated image processing techniques are crucial for addressing this.
- High-resolution imaging: Subtle cracks or small particulate matter often require high-resolution imaging for accurate detection. High-resolution cameras and image processing algorithms capable of handling large image files are necessary for capturing and analyzing these fine details. Improved image resolution directly improves the sensitivity and accuracy of defect detection.
- Developing standardized image acquisition protocols: Establishing standardized procedures for vial presentation, lighting, and image capture is vital for obtaining consistent and comparable images across different batches and inspection runs. These protocols must be meticulously documented and followed for regulatory compliance.
Defect Detection and Classification using AI and Image Processing
Once acquired, the images must be analyzed for defects. This is where artificial intelligence (AI) and advanced image processing algorithms play a crucial role. However, challenges remain:
- Developing robust algorithms: Creating algorithms capable of accurately identifying diverse defects (cracks, discoloration, particulate matter, cake collapse, vial breakage) in lyophilized products is complex. These algorithms must be robust enough to handle variations in product appearance and lighting conditions.
- Minimizing false positives and false negatives: A major challenge is balancing sensitivity and specificity. False positives (incorrectly identifying a non-defect as a defect) lead to unnecessary rejection of good products, while false negatives (missing actual defects) pose a significant risk to patient safety. Fine-tuning AI models and employing advanced image processing techniques can help to minimize both.
- Machine learning and deep learning: The application of machine learning (ML) and deep learning (DL) techniques offers significant potential for improving the accuracy and efficiency of defect detection. These methods can learn complex patterns and features from large datasets of images, enabling the identification of subtle defects that might be missed by traditional methods.
- Training AI models: Effective training of AI models requires large, high-quality datasets of images with accurately labeled defects. Creating these datasets requires considerable effort and expertise, including manual inspection and verification of defects by trained personnel.
- Ongoing model retraining: Manufacturing processes and product characteristics can change over time, requiring ongoing retraining of AI models to maintain accuracy. Regularly updating the model with new data ensures its continued effectiveness.
Addressing the Complexity of Lyophilized Product Defects
Lyophilized drug products exhibit unique defect types, adding complexity to automated visual inspection:
- Diverse defect types: Cake collapse, cracking, discoloration, particulate matter, and vial breakage all require distinct detection algorithms. The ability to differentiate between these various defect types is essential for accurate quality control.
- Subtle defect identification: Some defects, such as hairline cracks or minor discolorations, are subtle and require sophisticated image analysis techniques for detection. Advanced image processing algorithms and high-resolution imaging are crucial for identifying these subtle defects.
- Critical vs. non-critical defects: Differentiating between defects that pose a significant risk to product quality and those that are inconsequential is crucial for efficient quality control. Establishing clear criteria for classifying defects is essential for making informed decisions about product acceptance or rejection.
- Regulatory compliance: Establishing clear acceptance criteria for each defect type is paramount for regulatory compliance. These criteria must be aligned with relevant guidelines and regulations, such as those from the FDA.
Integration and Validation of Automated Visual Inspection Systems
Successfully implementing AVI requires seamless integration and rigorous validation:
- System integration: The AVI system must integrate seamlessly with existing manufacturing and quality control systems to ensure efficient data flow and traceability. This involves integrating the AVI system with existing software and hardware, including databases and reporting systems.
- Regulatory compliance: Validation of the AVI system to meet regulatory requirements (e.g., GMP, 21 CFR Part 11) is crucial for ensuring compliance and maintaining product quality. This involves rigorous testing and documentation to demonstrate the system's accuracy, reliability, and performance.
- Data integrity and traceability: Maintaining data integrity and ensuring complete traceability throughout the inspection process is essential for regulatory compliance and efficient troubleshooting. This includes secure data storage, audit trails, and robust data management systems.
- Quality management systems: Implementing robust quality management systems to monitor system performance and address any issues promptly is critical for maintaining the accuracy and reliability of the AVI system.
Conclusion
Automated visual inspection of lyophilized drug products presents substantial challenges, but advancements in image processing and artificial intelligence offer promising solutions. By addressing the hurdles in image acquisition, defect detection, and system integration, the pharmaceutical industry can significantly improve quality control and reduce the risk of releasing defective products. Further research and development in robust algorithms, standardized protocols, and seamless system integration are essential. Investing in advanced automated visual inspection techniques is crucial for maintaining the highest standards in pharmaceutical quality control. Embrace advanced automated visual inspection and elevate your lyophilized drug product quality.
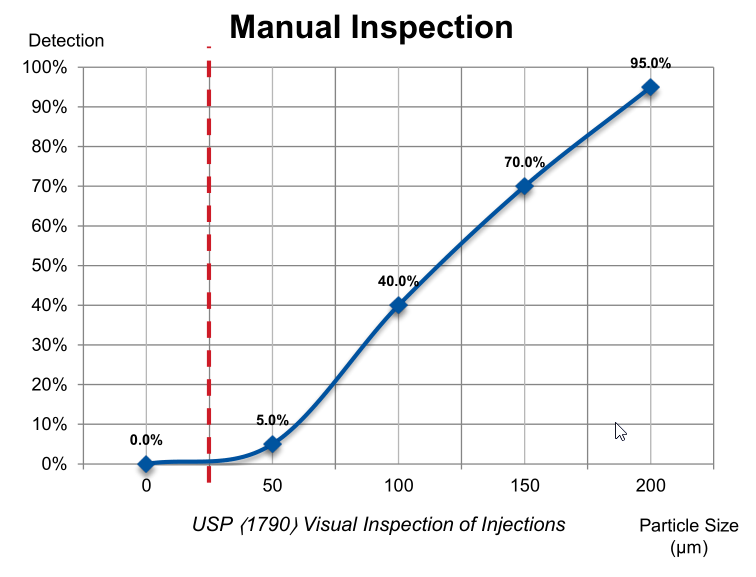
Featured Posts
-
Pope Leo I A Strong Message Against The Rise Of De Facto Atheism
May 11, 2025 -
Newark Mayor Arrested Outside Ice Detention Center Full Story
May 11, 2025 -
Genuine Representation Addressing The Needs Of Asian And Asian American Communities In Media
May 11, 2025 -
Comprehensive Senior Activities And Travel Calendar
May 11, 2025 -
El Inusual Regalo De Uruguay A China Un Avion Para El Crecimiento De Las Exportaciones Ganaderas
May 11, 2025