The Battle For Supremacy: How Google And OpenAI Differ In I/O And Io Strategies
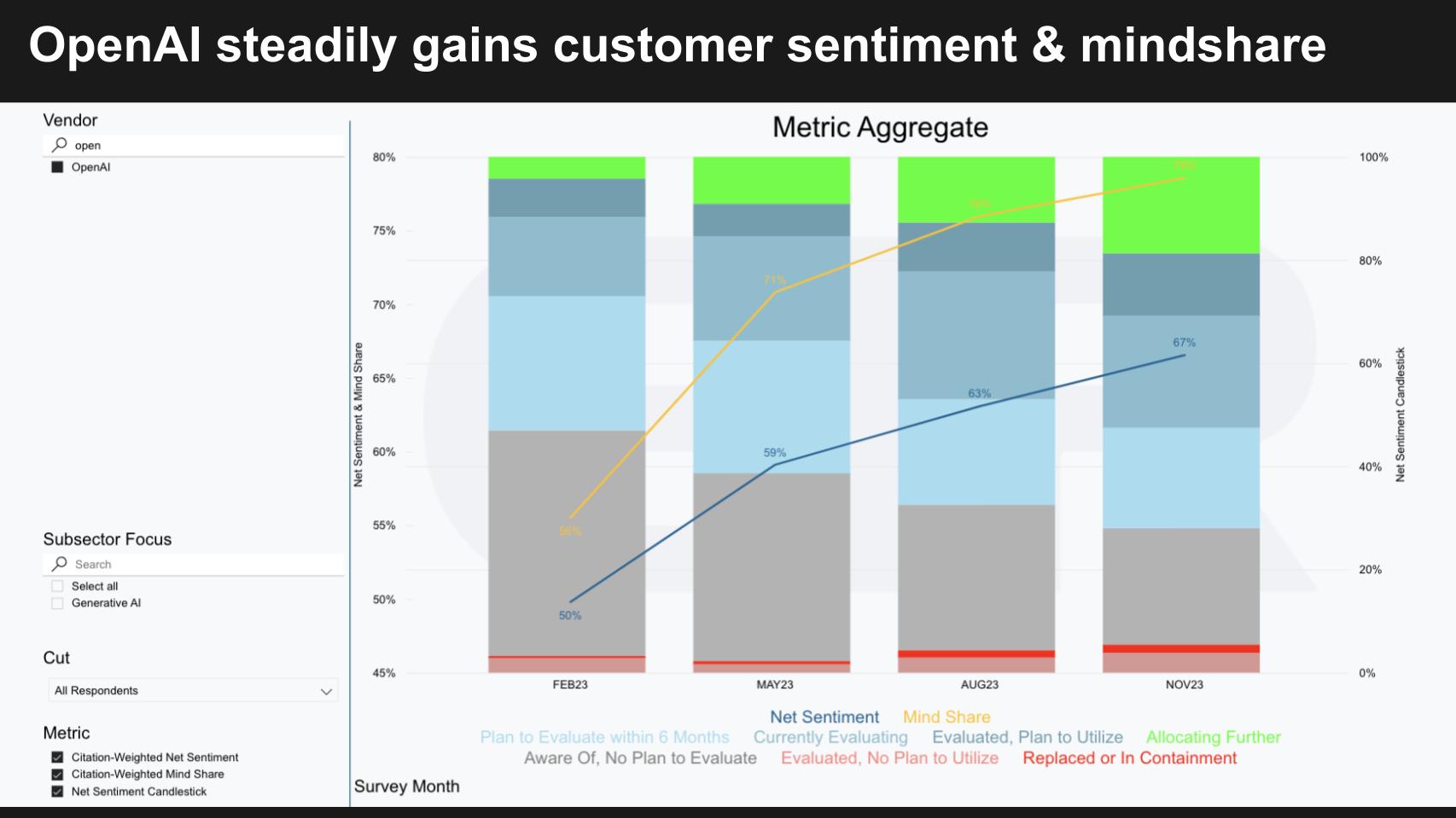
Table of Contents
Google's I/O and io Approach: A Focus on Scalability and Infrastructure
Google's dominance in AI is deeply rooted in its unparalleled infrastructure. Their I/O and io strategies are fundamentally built upon the scalability and reliability of their vast resources.
Google's Cloud Infrastructure and its Role in I/O
Google Cloud Platform (GCP) is the backbone of Google's I/O capabilities. Its extensive network of data centers and globally distributed resources allow for seamless handling of massive datasets and complex computations.
- Google Kubernetes Engine (GKE): Facilitates containerized deployments, ensuring scalability and efficient resource management for AI workloads.
- Google Compute Engine (GCE): Provides customizable virtual machines offering the flexibility to handle diverse AI model training and inference needs.
- Other relevant services: Cloud Storage, Cloud SQL, and Bigtable provide robust storage and database solutions crucial for efficient I/O operations.
GCP's infrastructure facilitates high-throughput data processing, crucial for training and deploying large-scale AI models. The global reach ensures low latency for users worldwide, a critical factor for real-time applications.
Google's Emphasis on Efficient Data Handling
Google invests heavily in optimizing data pipelines and I/O operations, particularly for massive datasets. This focus on efficiency underpins their ability to train and deploy complex AI models.
- TensorFlow: Google's open-source machine learning framework streamlines the data flow and model building process, minimizing latency and maximizing throughput.
- BigQuery: Google's serverless data warehouse provides fast querying of petabytes of data, crucial for feeding large AI models and enabling rapid insights.
These technologies contribute significantly to faster model training and inference times, allowing Google to consistently push the boundaries of AI capabilities. For example, TensorFlow's optimized operations minimize redundant data transfers, leading to significant speed improvements in training large language models.
OpenAI's I/O and io Strategy: Prioritizing Model Performance and Innovation
OpenAI's approach contrasts sharply with Google's. Instead of focusing primarily on infrastructure, OpenAI prioritizes developing cutting-edge AI models and making them easily accessible.
OpenAI's Model-Centric Approach
OpenAI’s strategy centers on pushing the limits of AI model performance. Their I/O considerations revolve around efficiently handling the enormous computational demands of these models and enabling smooth user interactions.
- GPT-3, DALL-E 2, and others: These models represent significant advancements in AI, requiring sophisticated I/O management to handle their vast parameter spaces and complex computations.
- Efficient model design: While computationally intensive, OpenAI designs its models with efficiency in mind, leveraging techniques to minimize unnecessary computations and optimize data flow within the model itself.
This model-centric strategy prioritizes innovation and pushing the boundaries of what's possible in AI, often at the cost of deploying models at the same scale as Google's.
OpenAI's API-First Strategy and its Impact on I/O
OpenAI's reliance on APIs for providing access to its models significantly impacts its I/O management. This approach makes its technology more accessible to developers and businesses but introduces its own set of challenges.
- Ease of integration: OpenAI's APIs offer a simple way to integrate powerful AI models into various applications.
- Scalability challenges: Managing the I/O demands of a large user base accessing the APIs requires robust infrastructure and efficient resource allocation.
OpenAI manages the I/O demands of their API through careful resource allocation and optimization techniques to ensure responsiveness and avoid bottlenecks, although their approach is less directly tied to owning the underlying infrastructure.
A Comparative Analysis: Google vs. OpenAI I/O Strategies
Comparing Google and OpenAI's I/O strategies reveals distinct strengths and weaknesses in their approaches.
Scalability and Infrastructure
Google's infrastructure-heavy approach provides unparalleled scalability and robustness. OpenAI's model-centric approach, while innovative, faces scalability challenges as model sizes and user demand increase.
- Google Strengths: Massive scalability, reliability, and global reach.
- Google Weaknesses: Higher upfront infrastructure investment.
- OpenAI Strengths: Focus on model innovation and leading-edge AI capabilities.
- OpenAI Weaknesses: Potential scalability bottlenecks depending on API usage.
The trade-offs between infrastructure investment and model performance optimization are significant considerations for both companies.
Cost and Accessibility
The cost and accessibility of each company's technologies differ significantly. Google's services are often geared towards enterprise-level users, while OpenAI's APIs offer more accessible options for individual developers and smaller businesses.
- Google: Typically higher costs, more suitable for large-scale deployments.
- OpenAI: More accessible pricing models, potentially lower costs for smaller projects.
These contrasting cost structures and target audiences influence the broader AI ecosystem, shaping the availability and adoption of different AI technologies.
Conclusion
Google and OpenAI's contrasting Google and OpenAI I/O and io strategies reflect their different priorities. Google emphasizes scalability and infrastructure, creating a robust foundation for large-scale AI deployments. OpenAI prioritizes model innovation and API accessibility, focusing on pushing the boundaries of AI capabilities and making them readily available to developers. Understanding these distinct approaches is crucial for navigating the rapidly evolving AI landscape. To further your understanding, explore the detailed documentation and resources available on both Google Cloud Platform and OpenAI's website. The ongoing evolution of Google and OpenAI I/O and io strategies will continue to shape the future of artificial intelligence.
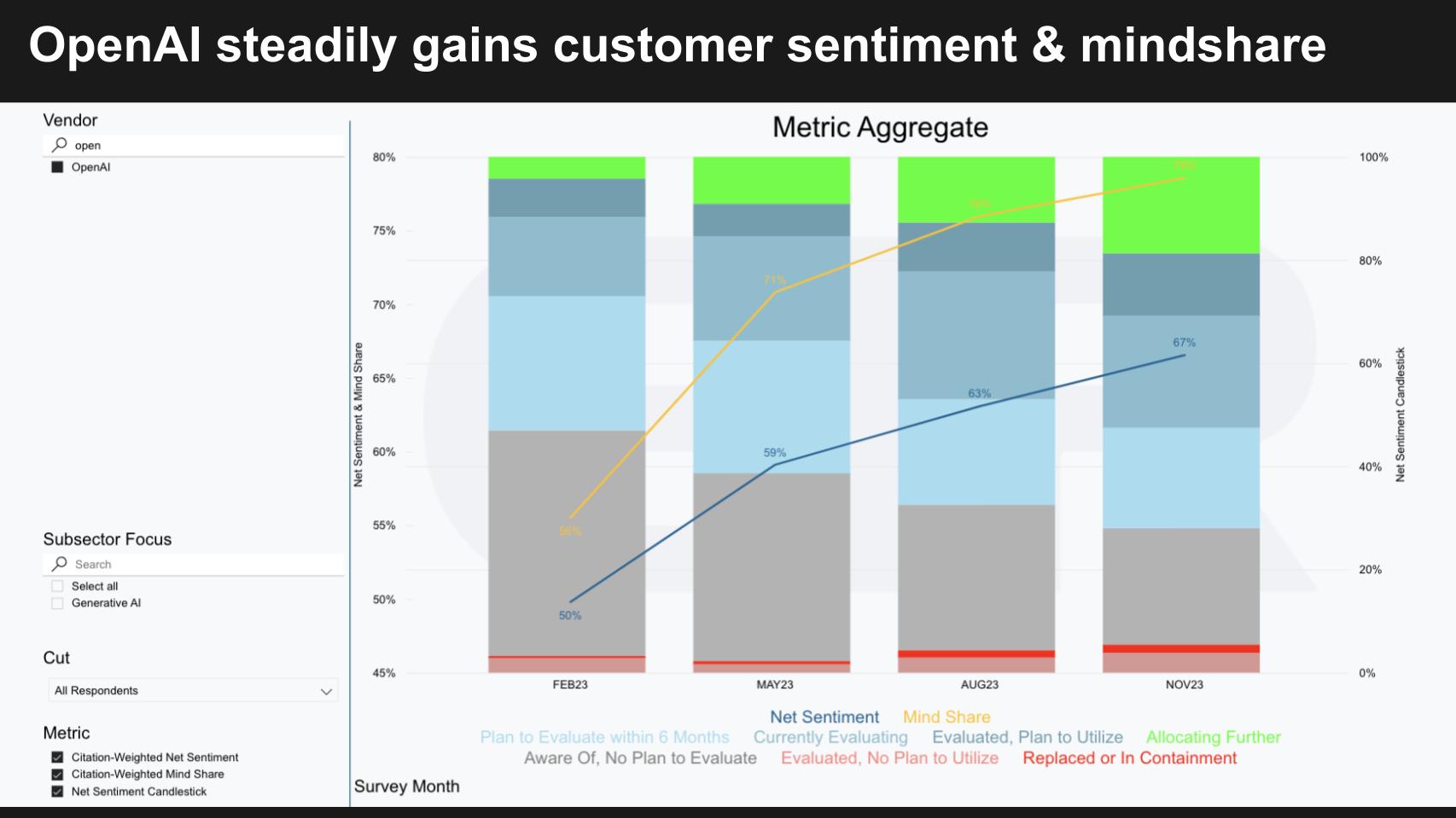
Featured Posts
-
Astmrar Almzahrat Fy Tl Abyb Llmtalbt Bieadt Alasra
May 26, 2025 -
Moto Gp Inggris 2025 Jadwal Lengkap Siaran Langsung Trans7 And Spotv Dan Klasemen
May 26, 2025 -
Analyzing The F1 Drivers Press Conference A Deep Dive
May 26, 2025 -
La Rtbf Et La Journee Mondiale Du Fact Checking Verifier L Information Pour Un Monde Meilleur
May 26, 2025 -
Pound Gains As Inflation Eases Boe Interest Rate Cut Speculation Falls
May 26, 2025
Latest Posts
-
Personal Loan Interest Rates Today Financing Starting Under 6
May 28, 2025 -
Finding A Direct Lender For Bad Credit Personal Loans Up To 5000
May 28, 2025 -
Personal Loans With Guaranteed Approval For Bad Credit Up To 5000
May 28, 2025 -
Abd Tueketici Kredileri Mart Ayi Artisinin Sebepleri Ve Sonuclari
May 28, 2025 -
Secure Personal Loans For Bad Credit Direct Lender Options And Up To 5000
May 28, 2025